Scaling up Machine Learning: Parallel and Distributed Approaches
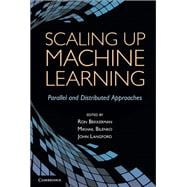
Scaling up Machine Learning: Parallel and Distributed Approaches
- ISBN 13:
9780521192248
- ISBN 10:
0521192242
- Format: Hardcover
- Copyright: 12/30/2011
- Publisher: Cambridge University Press
List Price $110.00 Save
TERM | PRICE | DUE |
---|---|---|
Free Shipping Both Ways
Highlight/Take Notes Like You Own It
Purchase/Extend Before Due Date
List Price $110.00 Save $1.10
Special Order: 1-2 Weeks
We Buy This Book Back!
Free Shipping On Every Order
Note: Supplemental materials are not guaranteed with Rental or Used book purchases.
Extend or Purchase Your Rental at Any Time
Need to keep your rental past your due date? At any time before your due date you can extend or purchase your rental through your account.